informatica
Tuesday, September 13, 2011
MAPPING PARAMETERS & VARIABLES
Mapping parameters and variables represent values in mappings and mapplets.
When we use a mapping parameter or variable in a mapping, first we declare the mapping parameter or variable for use in each mapplet or mapping. Then, we define a value for the mapping parameter or variable before we run the session.
MAPPING PARAMETERS
TIMESTAMP = $$IncludeDateTime
In the mapping, use a variable function to set the variable value to increment one day each time the session runs. If we set the initial value of $$IncludeDateTime to 8/1/2004, the first time the Integration Service runs the session, it reads only rows dated 8/1/2004. During the session, the Integration Service sets $$IncludeDateTime to 8/2/2004. It saves 8/2/2004 to the repository at the end of the session. The next time it runs the session, it reads only rows from August 2, 2004.
Used in following transformations:
When we declare a mapping parameter or variable in a mapping or a mapplet, we can enter an initial value. When the Integration Service needs an initial value, and we did not declare an initial value for the parameter or variable, the Integration Service uses a default value based on the data type of the parameter or variable.
Data ->Default Value
Numeric ->0
String ->Empty String
Date time ->1/1/1
Variable Values: Start value and current value of a mapping variable
Start Value:
The start value is the value of the variable at the start of the session. The Integration Service looks for the start value in the following order:
The current value is the value of the variable as the session progresses. When a session starts, the current value of a variable is the same as the start value. The final current value for a variable is saved to the repository at the end of a successful session. When a session fails to complete, the Integration Service does not update the value of the variable in the repository.
Note: If a variable function is not used to calculate the current value of a mapping variable, the start value of the variable is saved to the repository.
Variable Data type and Aggregation Type When we declare a mapping variable in a mapping, we need to configure the Data type and aggregation type for the variable. The IS uses the aggregate type of a Mapping variable to determine the final current value of the mapping variable.
Aggregation types are:
Variable functions determine how the Integration Service calculates the current value of a mapping variable in a pipeline.
SetMaxVariable: Sets the variable to the maximum value of a group of values. It ignores rows marked for update, delete, or reject. Aggregation type set to Max.
SetMinVariable: Sets the variable to the minimum value of a group of values. It ignores rows marked for update, delete, or reject. Aggregation type set to Min.
SetCountVariable: Increments the variable value by one. It adds one to the variable value when a row is marked for insertion, and subtracts one when the row is Marked for deletion. It ignores rows marked for update or reject. Aggregation type set to Count.
SetVariable: Sets the variable to the configured value. At the end of a session, it compares the final current value of the variable to the start value of the variable. Based on the aggregate type of the variable, it saves a final value to the repository.
Creating Mapping Parameters and Variables
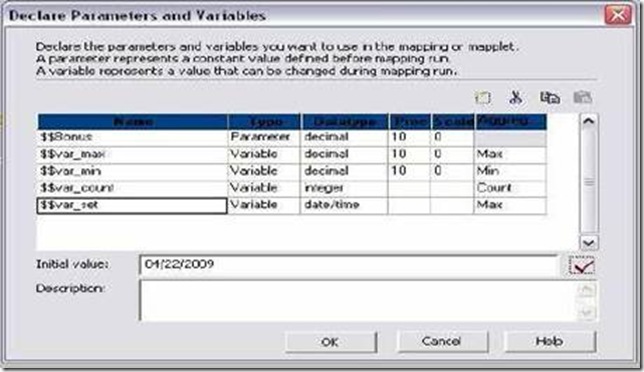
12. Create 5 output ports out_ TOTAL_SAL, out_MAX_VAR, out_MIN_VAR,
out_COUNT_VAR and out_SET_VAR.
13. Open expression editor for TOTAL_SAL. Do the same as we did earlier for SAL+ COMM. To add $$BONUS to it, select variable tab and select the parameter from mapping parameter. SAL + COMM + $$Bonus
14. Open Expression editor for out_max_var.
15. Select the variable function SETMAXVARIABLE from left side pane. Select
$$var_max from variable tab and SAL from ports tab as shown below.SETMAXVARIABLE($$var_max,SAL)
![clip_image002[5] clip_image002[5]](http://lh6.ggpht.com/_MbhSjEtmzI8/TanPkSZPLPI/AAAAAAAAAKg/L3Jc72Uym0Q/clip_image002%5B5%5D_thumb%5B3%5D.jpg?imgmax=800)
17. Open Expression editor for out_min_var and write the following expression:
SETMINVARIABLE($$var_min,SAL). Validate the expression.
18. Open Expression editor for out_count_var and write the following expression:
SETCOUNTVARIABLE($$var_count). Validate the expression.
19. Open Expression editor for out_set_var and write the following expression:
SETVARIABLE($$var_set,ADD_TO_DATE(HIREDATE,'MM',1)). Validate.
20. Click OK. Expression Transformation below:
21. Link all ports from expression to target and Validate Mapping and Save it.
22. See mapping picture on next page.
![clip_image002[9] clip_image002[9]](http://lh3.ggpht.com/_MbhSjEtmzI8/TanPnDAFk4I/AAAAAAAAAKw/LzjG-r7-UO0/clip_image002%5B9%5D_thumb%5B1%5D.jpg?imgmax=800)
PARAMETER FILE
Parameter files contain several sections preceded by a heading. The heading identifies the Integration Service, Integration Service process, workflow, worklet, or session to which we want to assign parameters or variables.
![clip_image002[11] clip_image002[11]](http://lh3.ggpht.com/_MbhSjEtmzI8/TanPoRjYOFI/AAAAAAAAAK4/nPDHIKuVhpo/clip_image002%5B11%5D_thumb%5B4%5D.jpg?imgmax=800)
Sample Parameter File for Our example:
In the parameter file, folder and session names are case sensitive.
Create a text file in notepad with name Para_File.txt
[Practice.ST:s_m_MP_MV_Example]
$$Bonus=1000
$$var_max=500
$$var_min=1200
$$var_count=0
CONFIGURING PARAMTER FILE
We can specify the parameter file name and directory in the workflow or session properties.
To enter a parameter file in the workflow properties:
1. Open a Workflow in the Workflow Manager.
2. Click Workflows > Edit.
3. Click the Properties tab.
4. Enter the parameter directory and name in the Parameter Filename field.
5. Click OK.
To enter a parameter file in the session properties:
1. Open a session in the Workflow Manager.
2. Click the Properties tab and open the General Options settings.
3. Enter the parameter directory and name in the Parameter Filename field.
4. Example: D:\Files\Para_File.txt or $PMSourceFileDir\Para_File.txt
5. Click OK.
When we use a mapping parameter or variable in a mapping, first we declare the mapping parameter or variable for use in each mapplet or mapping. Then, we define a value for the mapping parameter or variable before we run the session.
MAPPING PARAMETERS
- A mapping parameter represents a constant value that we can define before running a session.
- A mapping parameter retains the same value throughout the entire session.
- After we create a parameter, it appears in the Expression Editor.
- We can then use the parameter in any expression in the mapplet or mapping.
- We can also use parameters in a source qualifier filter, user-defined join, or extract override, and in the Expression Editor of reusable transformations.
- Unlike mapping parameters, mapping variables are values that can change between sessions.
- The Integration Service saves the latest value of a mapping variable to the repository at the end of each successful session.
- We can override a saved value with the parameter file.
- We can also clear all saved values for the session in the Workflow Manager.
TIMESTAMP = $$IncludeDateTime
In the mapping, use a variable function to set the variable value to increment one day each time the session runs. If we set the initial value of $$IncludeDateTime to 8/1/2004, the first time the Integration Service runs the session, it reads only rows dated 8/1/2004. During the session, the Integration Service sets $$IncludeDateTime to 8/2/2004. It saves 8/2/2004 to the repository at the end of the session. The next time it runs the session, it reads only rows from August 2, 2004.
Used in following transformations:
- Expression
- Filter
- Router
- Update Strategy
When we declare a mapping parameter or variable in a mapping or a mapplet, we can enter an initial value. When the Integration Service needs an initial value, and we did not declare an initial value for the parameter or variable, the Integration Service uses a default value based on the data type of the parameter or variable.
Data ->Default Value
Numeric ->0
String ->Empty String
Date time ->1/1/1
Variable Values: Start value and current value of a mapping variable
Start Value:
The start value is the value of the variable at the start of the session. The Integration Service looks for the start value in the following order:
- Value in parameter file
- Value saved in the repository
- Initial value
- Default value
The current value is the value of the variable as the session progresses. When a session starts, the current value of a variable is the same as the start value. The final current value for a variable is saved to the repository at the end of a successful session. When a session fails to complete, the Integration Service does not update the value of the variable in the repository.
Note: If a variable function is not used to calculate the current value of a mapping variable, the start value of the variable is saved to the repository.
Variable Data type and Aggregation Type When we declare a mapping variable in a mapping, we need to configure the Data type and aggregation type for the variable. The IS uses the aggregate type of a Mapping variable to determine the final current value of the mapping variable.
Aggregation types are:
- Count: Integer and small integer data types are valid only.
- Max: All transformation data types except binary data type are valid.
- Min: All transformation data types except binary data type are valid.
Variable functions determine how the Integration Service calculates the current value of a mapping variable in a pipeline.
SetMaxVariable: Sets the variable to the maximum value of a group of values. It ignores rows marked for update, delete, or reject. Aggregation type set to Max.
SetMinVariable: Sets the variable to the minimum value of a group of values. It ignores rows marked for update, delete, or reject. Aggregation type set to Min.
SetCountVariable: Increments the variable value by one. It adds one to the variable value when a row is marked for insertion, and subtracts one when the row is Marked for deletion. It ignores rows marked for update or reject. Aggregation type set to Count.
SetVariable: Sets the variable to the configured value. At the end of a session, it compares the final current value of the variable to the start value of the variable. Based on the aggregate type of the variable, it saves a final value to the repository.
Creating Mapping Parameters and Variables
- Open the folder where we want to create parameter or variable.
- In the Mapping Designer, click Mappings > Parameters and Variables. -or- In the Mapplet Designer, click Mapplet > Parameters and Variables.
- Click the add button.
- Enter name. Do not remove $$ from name.
- Select Type and Data type. Select Aggregation type for mapping variables.
- Give Initial Value. Click ok.
- EMP will be source table.
- Create a target table MP_MV_EXAMPLE having columns: EMPNO, ENAME, DEPTNO, TOTAL_SAL, MAX_VAR, MIN_VAR, COUNT_VAR and SET_VAR.
- TOTAL_SAL = SAL+ COMM + $$BONUS (Bonus is mapping parameter that changes every month)
- SET_VAR: We will be added one month to the HIREDATE of every employee.
- Create shortcuts as necessary.
- Open folder where we want to create the mapping.
- Click Tools -> Mapping Designer.
- Click Mapping-> Create-> Give name. Ex: m_mp_mv_example
- Drag EMP and target table.
- Transformation -> Create -> Select Expression for list -> Create –> Done.
- Drag EMPNO, ENAME, HIREDATE, SAL, COMM and DEPTNO to Expression.
- Create Parameter $$Bonus and Give initial value as 200.
- Create variable $$var_max of MAX aggregation type and initial value 1500.
- Create variable $$var_min of MIN aggregation type and initial value 1500.
- Create variable $$var_count of COUNT aggregation type and initial value 0. COUNT is visible when datatype is INT or SMALLINT.
- Create variable $$var_set of MAX aggregation type.
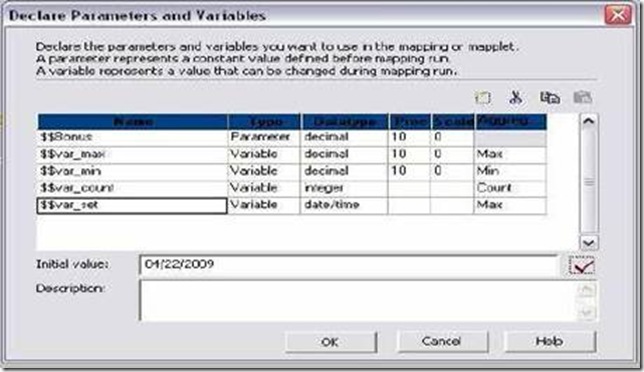
12. Create 5 output ports out_ TOTAL_SAL, out_MAX_VAR, out_MIN_VAR,
out_COUNT_VAR and out_SET_VAR.
13. Open expression editor for TOTAL_SAL. Do the same as we did earlier for SAL+ COMM. To add $$BONUS to it, select variable tab and select the parameter from mapping parameter. SAL + COMM + $$Bonus
14. Open Expression editor for out_max_var.
15. Select the variable function SETMAXVARIABLE from left side pane. Select
$$var_max from variable tab and SAL from ports tab as shown below.SETMAXVARIABLE($$var_max,SAL)
![clip_image002[5] clip_image002[5]](http://lh6.ggpht.com/_MbhSjEtmzI8/TanPkSZPLPI/AAAAAAAAAKg/L3Jc72Uym0Q/clip_image002%5B5%5D_thumb%5B3%5D.jpg?imgmax=800)
17. Open Expression editor for out_min_var and write the following expression:
SETMINVARIABLE($$var_min,SAL). Validate the expression.
18. Open Expression editor for out_count_var and write the following expression:
SETCOUNTVARIABLE($$var_count). Validate the expression.
19. Open Expression editor for out_set_var and write the following expression:
SETVARIABLE($$var_set,ADD_TO_DATE(HIREDATE,'MM',1)). Validate.
20. Click OK. Expression Transformation below:
![clip_image002[7] clip_image002[7]](http://lh6.ggpht.com/_MbhSjEtmzI8/TanPlzc1mPI/AAAAAAAAAKo/KYpOuwOVo9Y/clip_image002%5B7%5D_thumb%5B4%5D.jpg?imgmax=800)
21. Link all ports from expression to target and Validate Mapping and Save it.
22. See mapping picture on next page.
![clip_image002[9] clip_image002[9]](http://lh3.ggpht.com/_MbhSjEtmzI8/TanPnDAFk4I/AAAAAAAAAKw/LzjG-r7-UO0/clip_image002%5B9%5D_thumb%5B1%5D.jpg?imgmax=800)
PARAMETER FILE
- A parameter file is a list of parameters and associated values for a workflow, worklet, or session.
- Parameter files provide flexibility to change these variables each time we run a workflow or session.
- We can create multiple parameter files and change the file we use for a session or workflow. We can create a parameter file using a text editor such as WordPad or Notepad.
- Enter the parameter file name and directory in the workflow or session properties.
- Workflow variable: References values and records information in a workflow.
- Worklet variable: References values and records information in a worklet. Use predefined worklet variables in a parent workflow, but we cannot use workflow variables from the parent workflow in a worklet.
- Session parameter: Defines a value that can change from session to session, such as a database connection or file name.
- Mapping parameter and Mapping variable
Parameter files contain several sections preceded by a heading. The heading identifies the Integration Service, Integration Service process, workflow, worklet, or session to which we want to assign parameters or variables.
- Make session and workflow.
- Give connection information for source and target table.
- Run workflow and see result.
![clip_image002[11] clip_image002[11]](http://lh3.ggpht.com/_MbhSjEtmzI8/TanPoRjYOFI/AAAAAAAAAK4/nPDHIKuVhpo/clip_image002%5B11%5D_thumb%5B4%5D.jpg?imgmax=800)
Sample Parameter File for Our example:
In the parameter file, folder and session names are case sensitive.
Create a text file in notepad with name Para_File.txt
[Practice.ST:s_m_MP_MV_Example]
$$Bonus=1000
$$var_max=500
$$var_min=1200
$$var_count=0
CONFIGURING PARAMTER FILE
We can specify the parameter file name and directory in the workflow or session properties.
To enter a parameter file in the workflow properties:
1. Open a Workflow in the Workflow Manager.
2. Click Workflows > Edit.
3. Click the Properties tab.
4. Enter the parameter directory and name in the Parameter Filename field.
5. Click OK.
To enter a parameter file in the session properties:
1. Open a session in the Workflow Manager.
2. Click the Properties tab and open the General Options settings.
3. Enter the parameter directory and name in the Parameter Filename field.
4. Example: D:\Files\Para_File.txt or $PMSourceFileDir\Para_File.txt
5. Click OK.
Monday, September 12, 2011
Informatica Dynamic Lookup Cache
A LookUp cache does not change once built. But what if the underlying lookup table changes the data after the lookup cache is created? Is there a way so that the cache always remain up-to-date even if the underlying table changes?
Dynamic Lookup Cache
Let's think about this scenario. You are loading your target table through a mapping. Inside the mapping you have a Lookup and in the Lookup, you are actually looking up the same target table you are loading. You may ask me, "So? What's the big deal? We all do it quite often...". And yes you are right. There is no "big deal" because Informatica (generally) caches the lookup table in the very beginning of the mapping, so whatever record getting inserted to the target table through the mapping, will have no effect on the Lookup cache. The lookup will still hold the previously cached data, even if the underlying target table is changing.
But what if you want your Lookup cache to get updated as and when the target table is changing? What if you want your lookup cache to always show the exact snapshot of the data in your target table at that point in time? Clearly this requirement will not be fullfilled in case you use a static cache. You will need a dynamic cache to handle this.
But, What if you had a flat file as source with many duplicate records?
Would the scenario be same? No, see the below illustration.
Here are some more examples when you may consider using dynamic lookup,
Notice that Integration Service actually flags the rows based on the above three conditions.
And that's a great thing, because, if you know the flag you can actually reroute the row to achieve different logic. This flag port is called
Oh, forgot to tell you the actual values that you can expect in NewLookupRow port are:
And here I provide you the screenshot of the lookup below. Lookup ports screen shot first,
Image: Dynamic Lookup Ports Tab

And here is Dynamic Lookup Properties Tab
If you check the mapping screenshot, there I have used a router to reroute the INSERT group and UPDATE group. The router screenshot is also given below. New records are routed to the INSERT group and existing records are routed to the UPDATE group.
Router Transformation Groups Tab
When we select Sequence-ID in the Associated Port column, the Integration Service generates a sequence ID for each row it inserts into the lookup cache.
When the Integration Service creates the dynamic lookup cache, it tracks the range of values in the cache associated with any port using a sequence ID and it generates a key for the port by incrementing the greatest sequence ID existing value by one, when the inserting a new row of data into the cache.
When the Integration Service reaches the maximum number for a generated sequence ID, it starts over at one and increments each sequence ID by one until it reaches the smallest existing value minus one. If the Integration Service runs out of unique sequence ID numbers, the session fails.
When you update a dynamic lookup cache and target table, the source data might contain some null values. The Integration Service can handle the null values in the following ways:
When we choose to ignore NULLs, we must verify that we output the same values to the target that the Integration Service writes to the lookup cache. We can Configure the mapping based on the value we want the Integration Service to output from the lookup/output ports when it updates a row in the cache, so that lookup cache and the target table might not become unsynchronized
When we run a session that uses a dynamic lookup cache, the Integration Service compares the values in all lookup ports with the values in their associated input ports by default.
It compares the values to determine whether or not to update the row in the lookup cache. When a value in an input port differs from the value in the lookup port, the Integration Service updates the row in the cache.
But what if we don't want to compare all ports? We can choose the ports we want the Integration Service to ignore when it compares ports. The Designer only enables this property for lookup/output ports when the port is not used in the lookup condition. We can improve performance by ignoring some ports during comparison.
We might want to do this when the source data includes a column that indicates whether or not the row contains data we need to update. Select the Ignore in Comparison property for all lookup ports except the port that indicates whether or not to update the row in the cache and target table.
Note: We must configure the Lookup transformation to compare at least one port else the Integration Service fails the session when we ignore all ports.

But what if you want your Lookup cache to get updated as and when the target table is changing? What if you want your lookup cache to always show the exact snapshot of the data in your target table at that point in time? Clearly this requirement will not be fullfilled in case you use a static cache. You will need a dynamic cache to handle this.
But why anyone will need a dynamic cache?
To understand this, let's first understand a static cache scenarioStatic Cache Scenario
Let's suppose you run a retail business and maintain all your customer information in a customer master table (RDBMS table). Every night, all the customers from your customer master table is loaded in to a Customer Dimension table in your data warehouse. Your source customer table is a transaction system table, probably in 3rd normal form, and does not store history. Meaning, if a customer changes his address, the old address is updated with the new address. But your data warehouse table stores the history (may be in the form of SCD Type-II). There is a map that loads your data warehouse table from the source table. Typically you do a Lookup on target (static cache) and check with your every incoming customer record to determine if the customer is already existing in target or not. If the customer is not already existing in target, you conclude the customer is new and INSERT the record whereas if the customer is already existing, you may want to update the target record with this new record (if the record is updated). This is illustrated below, You don't need dynamic Lookup cache for this
Image: A static Lookup Cache to determine if a source record is new or updatable
Dynamic Lookup Cache Scenario
Notice in the previous example I mentioned that your source table is an RDBMS table. This ensures that your source table does not have any duplicate record.But, What if you had a flat file as source with many duplicate records?
Would the scenario be same? No, see the below illustration.

Image: A Scenario illustrating the use of dynamic lookup cache
Here are some more examples when you may consider using dynamic lookup,
- Updating a master customer table with both new and updated customer information coming together as shown above
- Loading data into a slowly changing dimension table and a fact table at the same time. Remember, you typically lookup the dimension while loading to fact. So you load dimension table before loading fact table. But using dynamic lookup, you can load both simultaneously.
- Loading data from a file with many duplicate records and to eliminate duplicate records in target by updating a duplicate row i.e. keeping the most recent row or the initial row
- Loading the same data from multiple sources using a single mapping. Just consider the previous Retail business example. If you have more than one shops and Linda has visited two of your shops for the first time, customer record Linda will come twice during the same load.
So, How does dynamic lookup work?
When the Integration Service reads a row from the source, it updates the lookup cache by performing one of the following actions:- Inserts the row into the cache: If the incoming row is not in the cache, the Integration Service inserts the row in the cache based on input ports or generated Sequence-ID. The Integration Service flags the row as insert.
- Updates the row in the cache: If the row exists in the cache, the Integration Service updates the row in the cache based on the input ports. The Integration Service flags the row as update.
- Makes no change to the cache: This happens when the row exists in the cache and the lookup is configured or specified To Insert New Rows only or, the row is not in the cache and lookup is configured to update existing rows only or, the row is in the cache, but based on the lookup condition, nothing changes. The Integration Service flags the row as unchanged.
Notice that Integration Service actually flags the rows based on the above three conditions.
And that's a great thing, because, if you know the flag you can actually reroute the row to achieve different logic. This flag port is called
- NewLookupRow
Oh, forgot to tell you the actual values that you can expect in NewLookupRow port are:
- 0 = Integration Service does not update or insert the row in the cache.
- 1 = Integration Service inserts the row into the cache.
- 2 = Integration Service updates the row in the cache.
Example of Dynamic Lookup Implementation
Ok, I design a mapping for you to show Dynamic lookup implementation. I have given a full screenshot of the mapping. Since the screenshot is slightly bigger, so I link it below. Just click to expand the image.And here I provide you the screenshot of the lookup below. Lookup ports screen shot first,


And here is Dynamic Lookup Properties Tab

If you check the mapping screenshot, there I have used a router to reroute the INSERT group and UPDATE group. The router screenshot is also given below. New records are routed to the INSERT group and existing records are routed to the UPDATE group.

Router Transformation Groups Tab
About the Sequence-ID
While using a dynamic lookup cache, we must associate each lookup/output port with an input/output port or a sequence ID. The Integration Service uses the data in the associated port to insert or update rows in the lookup cache. The Designer associates the input/output ports with the lookup/output ports used in the lookup condition.When we select Sequence-ID in the Associated Port column, the Integration Service generates a sequence ID for each row it inserts into the lookup cache.
When the Integration Service creates the dynamic lookup cache, it tracks the range of values in the cache associated with any port using a sequence ID and it generates a key for the port by incrementing the greatest sequence ID existing value by one, when the inserting a new row of data into the cache.
When the Integration Service reaches the maximum number for a generated sequence ID, it starts over at one and increments each sequence ID by one until it reaches the smallest existing value minus one. If the Integration Service runs out of unique sequence ID numbers, the session fails.
About the Dynamic Lookup Output Port
The lookup/output port output value depends on whether we choose to output old or new values when the Integration Service updates a row:- Output old values on update: The Integration Service outputs the value that existed in the cache before it updated the row.
- Output new values on update: The Integration Service outputs the updated value that it writes in the cache. The lookup/output port value matches the input/output port value.
Handling NULL in dynamic LookUp
If the input value is NULL and we select the Ignore Null inputs for Update property for the associated input port, the input value does not equal the lookup value or the value out of the input/output port. When you select the Ignore Null property, the lookup cache and the target table might become unsynchronized if you pass null values to the target. You must verify that you do not pass null values to the target.When you update a dynamic lookup cache and target table, the source data might contain some null values. The Integration Service can handle the null values in the following ways:
- Insert null values: The Integration Service uses null values from the source and updates the lookup cache and target table using all values from the source.
- Ignore Null inputs for Update property : The Integration Service ignores the null values in the source and updates the lookup cache and target table using only the not null values from the source.
When we choose to ignore NULLs, we must verify that we output the same values to the target that the Integration Service writes to the lookup cache. We can Configure the mapping based on the value we want the Integration Service to output from the lookup/output ports when it updates a row in the cache, so that lookup cache and the target table might not become unsynchronized
- New values. Connect only lookup/output ports from the Lookup transformation to the target.
- Old values. Add an Expression transformation after the Lookup transformation and before the Filter or Router transformation. Add output ports in the Expression transformation for each port in the target table and create expressions to ensure that we do not output null input values to the target.
When we run a session that uses a dynamic lookup cache, the Integration Service compares the values in all lookup ports with the values in their associated input ports by default.
It compares the values to determine whether or not to update the row in the lookup cache. When a value in an input port differs from the value in the lookup port, the Integration Service updates the row in the cache.
But what if we don't want to compare all ports? We can choose the ports we want the Integration Service to ignore when it compares ports. The Designer only enables this property for lookup/output ports when the port is not used in the lookup condition. We can improve performance by ignoring some ports during comparison.
We might want to do this when the source data includes a column that indicates whether or not the row contains data we need to update. Select the Ignore in Comparison property for all lookup ports except the port that indicates whether or not to update the row in the cache and target table.
Note: We must configure the Lookup transformation to compare at least one port else the Integration Service fails the session when we ignore all ports.
Sunday, September 11, 2011
All about Informatica Lookup
A Lookup is a Passive , Connected or Unconnected Transformation used to look up data in a relational table, view, synonym or flat file. The integration service queries the lookup table to retrieve a value based on the input source value and the lookup condition.
Lookup can be Cached or Uncached . If we cache the lookup then again we can further go for static or dynamic or persistent cache,named cache or unnamed cache . By default lookup transformations are cached and static.
Lookup Ports Tab
The Ports tab of Lookup Transformation contains
Input Ports: Create an input port for each lookup port we want to use in the lookup condition. We must have at least one input or input/output port in a lookup transformation.
Output Ports: Create an output port for each lookup port we want to link to another transformation. For connected lookups, we must have at least one output port. For unconnected lookups, we must select a lookup port as a return port (R) to pass a return value.
Lookup Port: The Designer designates each column of the lookup source as a lookup port.
Return Port: An unconnected Lookup transformation has one return port that returns one column of data to the calling transformation through this port.
Notes: We can delete lookup ports from a relational lookup if the mapping does not use the lookup ports which will give us performance gain. But if the lookup source is a flat file then deleting of lookup ports fails the session.
Now let us have a look on the Properties Tab of the Lookup Transformation:
Lookup Sql Override: Override the default SQL statement to add a WHERE clause or to join multiple tables.
Lookup table name: The base table on which the lookup is performed.
Lookup Source Filter: We can apply filter conditions on the lookup table so as to reduce the number of records. For example, we may want to select the active records of the lookup table hence we may use the condition CUSTOMER_DIM.ACTIVE_FLAG = 'Y'.
Lookup caching enabled: If option is checked it caches the lookup table during the session run. Otherwise it goes for uncached relational database hit. Remember to implement database index on the columns used in the lookup condition to provide better performance when the lookup in Uncached.
Lookup policy on multiple match: While lookup if the integration service finds multiple match we can configure the lookup to return the First Value, Last Value, Any Value or to Report Error.
Lookup condition: The condition to lookup values from the lookup table based on source input data. For example, IN_EmpNo=EmpNo.
Connection Information: Query the lookup table from the source or target connection. In can of flat file lookup we can give the file path and name, whether direct or indirect.
Source Type: Determines whether the source is relational database or flat file.
Tracing Level: It provides the amount of detail in the session log for the transformation. Options available are Normal, Terse, Vebose Initialization, Verbose Data.
Lookup cache directory name: Determines the directory name where the lookup cache files will reside.
Lookup cache persistent: Indicates whether we are going for persistent cache or non-persistent cache.
Dynamic Lookup Cache: When checked We are going for Dyanamic lookup cache else static lookup cache is used.
Output Old Value On Update: Defines whether the old value for output ports will be used to update an existing row in dynamic cache.
Cache File Name Prefix: Lookup will used this named persistent cache file based on the base lookup table.
Re-cache from lookup source: When checked, integration service rebuilds lookup cache from lookup source when the lookup instance is called in the session.
Insert Else Update: Insert the record if not found in cache, else update it. Option is available when using dynamic lookup cache.
Update Else Insert: Update the record if found in cache, else insert it. Option is available when using dynamic lookup cache.
Datetime Format: Used when source type is file to determine the date and time format of lookup columns.
Thousand Separator: By default it is None, used when source type is file to determine the thousand separator.
Decimal Separator: By default it is "." else we can use "," and used when source type is file to determine the thousand separator.
Case Sensitive String Comparison: To be checked when we want to go for Case sensitive String values in lookup comparison. Used when source type is file.
Null ordering: Determines whether NULL is the highest or lowest value. Used when source type is file.
Sorted Input: Checked whenever we expect the input data to be sorted and is used when the source type is flat file.
Lookup source is static: When checked it assumes that the lookup source is not going to change during the session run.
Pre-build lookup cache: Default option is Auto. If we want the integration service to start building the cache whenever the session just begins we can chose the option Always allowed.
All about Informatica LookUp Transformation
A connected lookup recieves source data, performs a lookup and returns data to the pipeline; While an unconnected lookup is not connected to source or target and is called by a transformation in the pipeline by :LKP expression which in turn returns only one column value to the calling transformation.Lookup can be Cached or Uncached . If we cache the lookup then again we can further go for static or dynamic or persistent cache,named cache or unnamed cache . By default lookup transformations are cached and static.
Lookup Ports Tab
The Ports tab of Lookup Transformation contains
Input Ports: Create an input port for each lookup port we want to use in the lookup condition. We must have at least one input or input/output port in a lookup transformation.
Output Ports: Create an output port for each lookup port we want to link to another transformation. For connected lookups, we must have at least one output port. For unconnected lookups, we must select a lookup port as a return port (R) to pass a return value.
Lookup Port: The Designer designates each column of the lookup source as a lookup port.
Return Port: An unconnected Lookup transformation has one return port that returns one column of data to the calling transformation through this port.
Notes: We can delete lookup ports from a relational lookup if the mapping does not use the lookup ports which will give us performance gain. But if the lookup source is a flat file then deleting of lookup ports fails the session.
Now let us have a look on the Properties Tab of the Lookup Transformation:
Lookup Sql Override: Override the default SQL statement to add a WHERE clause or to join multiple tables.
Lookup table name: The base table on which the lookup is performed.
Lookup Source Filter: We can apply filter conditions on the lookup table so as to reduce the number of records. For example, we may want to select the active records of the lookup table hence we may use the condition CUSTOMER_DIM.ACTIVE_FLAG = 'Y'.
Lookup caching enabled: If option is checked it caches the lookup table during the session run. Otherwise it goes for uncached relational database hit. Remember to implement database index on the columns used in the lookup condition to provide better performance when the lookup in Uncached.
Lookup policy on multiple match: While lookup if the integration service finds multiple match we can configure the lookup to return the First Value, Last Value, Any Value or to Report Error.
Lookup condition: The condition to lookup values from the lookup table based on source input data. For example, IN_EmpNo=EmpNo.
Connection Information: Query the lookup table from the source or target connection. In can of flat file lookup we can give the file path and name, whether direct or indirect.
Source Type: Determines whether the source is relational database or flat file.
Tracing Level: It provides the amount of detail in the session log for the transformation. Options available are Normal, Terse, Vebose Initialization, Verbose Data.
Lookup cache directory name: Determines the directory name where the lookup cache files will reside.
Lookup cache persistent: Indicates whether we are going for persistent cache or non-persistent cache.
Dynamic Lookup Cache: When checked We are going for Dyanamic lookup cache else static lookup cache is used.
Output Old Value On Update: Defines whether the old value for output ports will be used to update an existing row in dynamic cache.
Cache File Name Prefix: Lookup will used this named persistent cache file based on the base lookup table.
Re-cache from lookup source: When checked, integration service rebuilds lookup cache from lookup source when the lookup instance is called in the session.
Insert Else Update: Insert the record if not found in cache, else update it. Option is available when using dynamic lookup cache.
Update Else Insert: Update the record if found in cache, else insert it. Option is available when using dynamic lookup cache.
Datetime Format: Used when source type is file to determine the date and time format of lookup columns.
Thousand Separator: By default it is None, used when source type is file to determine the thousand separator.
Decimal Separator: By default it is "." else we can use "," and used when source type is file to determine the thousand separator.
Case Sensitive String Comparison: To be checked when we want to go for Case sensitive String values in lookup comparison. Used when source type is file.
Null ordering: Determines whether NULL is the highest or lowest value. Used when source type is file.
Sorted Input: Checked whenever we expect the input data to be sorted and is used when the source type is flat file.
Lookup source is static: When checked it assumes that the lookup source is not going to change during the session run.
Pre-build lookup cache: Default option is Auto. If we want the integration service to start building the cache whenever the session just begins we can chose the option Always allowed.
Enterprise Data Warehouse Data Reconciliation Methodology
An enterprise data warehouse often fetches records from several disparate systems and store them centrally in an enterprise-wide warehouse. But what is the guarantee that the quality of data will not degrade in the process of centralization?
Many of the data warehouses are built on n-tier architecture with multiple data extraction and data insertion jobs between two consecutive tiers. As it happens, the nature of the data changes as it passes from one tier to the next tier. Data reconciliation is the method of reconciling or tie-up the data between any two consecutive tiers (layers).
One of the major reasons of information loss is loading failures or errors during loading. Such errors can occur due to several reasons e.g.
Inconsistent or non coherent data from source
Non-integrating data among different sources
Unclean/ non-profiled data
Un-handled exceptions
Constraint violations
Logical issues/ Inherent flaws in program
Technical failures like loss of connectivity, loss over network, space issue etc.
Failure due to any such issue can result into potential information loss leading to unreliable data quality for business process decision making.
Further more, if such issues are not rectified at the earliest, this becomes even more costly to “patch” later. Therefore this is highly suggested that a proper data reconciliation process must be in place in any data Extraction-Transformation-Load (ETL) process.
While data reconciliation may be a part of data quality assurance, these two things are not necessarily same.
Scope of data reconciliation should be limited to identify, if at all, there is any issue in the data or not. The scope should not be extended to automate the process of data investigation and pin pointing the issues.
A successful reconciliation process should only indicate whether or not the data is correct. It will not indicate why the data is not correct. Reconciliation process answers “what” part of the question, not “why” part of the question
In one business process, “customer”, “products”, “employee” etc. are some good example of master data. Ensuring the total number of customer in the source systems match exactly with the total number of customers in the target system is an example of customer master data reconciliation.
Some of the common examples of master data reconciliation can be the following measures,
1.Total count of rows, example
a. Total Customer in source and target
b. Total number of Products in source and target etc.
2.Total count of rows based on a condition, example
a. Total number of active customers
b. Total number of inactive customers etc.
Transactional data reconciliation is always done in terms of total sum. This prevents any mismatch otherwise caused due to varying granularity of qualifying dimensions. Also this total sum can be done on either full data or only on incremental data set.
Some examples measures used for transactional data reconciliation can be
1. Sum of total revenue calculated from source and target
2. Sum of total product sold calculated from source and target etc.
Data Reconciliation
Why Reconciliation is required?
In the process of extracting data from one source and then transforming the data and loading it to the next layer, the whole nature of the data can change considerably. It might also happen that some information is lost while transforming the data. A reconciliation process helps to identify such loss of information.One of the major reasons of information loss is loading failures or errors during loading. Such errors can occur due to several reasons e.g.
Inconsistent or non coherent data from source
Non-integrating data among different sources
Unclean/ non-profiled data
Un-handled exceptions
Constraint violations
Logical issues/ Inherent flaws in program
Technical failures like loss of connectivity, loss over network, space issue etc.
Failure due to any such issue can result into potential information loss leading to unreliable data quality for business process decision making.
Further more, if such issues are not rectified at the earliest, this becomes even more costly to “patch” later. Therefore this is highly suggested that a proper data reconciliation process must be in place in any data Extraction-Transformation-Load (ETL) process.
Scope of Data Reconciliation
Data reconciliation is often confused with the process of data quality testing. Even worse, sometimes data reconciliation process is used to investigate and pin point the data issues.While data reconciliation may be a part of data quality assurance, these two things are not necessarily same.
Scope of data reconciliation should be limited to identify, if at all, there is any issue in the data or not. The scope should not be extended to automate the process of data investigation and pin pointing the issues.
A successful reconciliation process should only indicate whether or not the data is correct. It will not indicate why the data is not correct. Reconciliation process answers “what” part of the question, not “why” part of the question
Methods of Data Reconciliation
Master Data Reconciliation
Master data reconciliation is the method of reconciling only the master data between source and target. Master data are generally unchanging or slowly changing in nature and no aggregation operation is done on the dataset. That is - the granularity of the data remains same in both source and target. That is why master data reconciliation is often relatively easy and quicker to implement.In one business process, “customer”, “products”, “employee” etc. are some good example of master data. Ensuring the total number of customer in the source systems match exactly with the total number of customers in the target system is an example of customer master data reconciliation.
Some of the common examples of master data reconciliation can be the following measures,
1.Total count of rows, example
a. Total Customer in source and target
b. Total number of Products in source and target etc.
2.Total count of rows based on a condition, example
a. Total number of active customers
b. Total number of inactive customers etc.
Transactional Data Reconciliation
Sales quantity, revenue, tax amount, service usage etc. are examples of transactional data. Transactional data make the very base of BI reports so any mismatch in transactional data can cause direct impact on the reliability of the report and the whole BI system in general. That is why reconciliation mechanism must be in-place in order to detect such a discrepancy before hand (meaning, before the data reach to the final business users)Transactional data reconciliation is always done in terms of total sum. This prevents any mismatch otherwise caused due to varying granularity of qualifying dimensions. Also this total sum can be done on either full data or only on incremental data set.
Some examples measures used for transactional data reconciliation can be
1. Sum of total revenue calculated from source and target
2. Sum of total product sold calculated from source and target etc.